如何用 OpenVINO™ 在本地快速部署 Llama 3.2
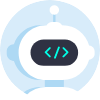
作者 | 武卓 英特尔 AI 软件布道师
随着 Llama 3.2 的刚刚发布,最新的AI模型进展比以往更加易于获取。借助 OpenVINO™ 和 Optimum Intel 的无缝集成,你可以在本地 Intel 硬件上压缩、优化并运行这个强大的模型。在本指南中,我们将带你完成整个流程,从环境搭建到最终执行,帮助你以最少的努力充分发挥 Llama 3.2 的潜力。
第0步:为开发准备你的机器!
对于第一次使用的用户,建议你按照Wiki中的基本设置步骤(1、2和3)进行。
机器设置并准备就绪 =)
第1步:下载 OpenVINO™ GenAI 示例代码
使用 OpenVINO™ GenAI API 在 Windows 上运行 Llama 3.1 的最简单方法是使用提供的示例代码进行设置。
首先,克隆仓库:
git clone https://github.com/openvinotoolkit/openvino.genai.git
在仓库中,你会找到一个名为 chat_sample 的 Python 示例。这个简洁的示例可以让你用不到40行代码执行 Llama 3.2,并与用户进行对话。它是开始探索模型功能的简便途径。
OpenVINO™ GenAI 的 Python 示例中的聊天示例
下面是示例代码的预览:
#!/usr/bin/env python3
# Copyright (C) 2024 Intel Corporation
# SPDX-License-Identifier: Apache-2.0
import argparse
import openvino_genai
def streamer(subword):
print(subword, end='', flush=True)
# Return flag corresponds whether generation should be stopped.
# False means continue generation.
return False
def main():
parser = argparse.ArgumentParser()
parser.add_argument('model_dir')
args = parser.parse_args()
device = 'CPU' # GPU can be used as well
pipe = openvino_genai.LLMPipeline(arg***odel_dir, device)
config = openvino_genai.GenerationConfig()
config.max_new_tokens = 100
pipe.start_chat()
while True:
prompt = input('question:\n')
if 'Stop!' == prompt:
break
pipe.generate(prompt, config, streamer)
print('\n----------')
pipe.finish_chat()
if '__main__' == __name__:
main()
接下来,让我们设置环境以处理模型的下载、转换和执行。
第2步:安装最新版本和依赖项
为了避免依赖冲突,建议创建一个单独的虚拟环境:
python -m venv openvino_venv
激活环境,
openvino_venv\Script\activate
现在,安装必要的依赖项:
python -m pip install --upgrade pip
pip install -U --pre openvino-genai openvino openvino-tokenizers[transformers] --extra-index-url https://storage.openvinotoolkit.org/simple/wheels/nightly
pip install --extra-index-url https://download.pytorch.org/whl/cpu "git+https://github.com/huggingface/optimum-intel.git" "git+https://github.com/openvinotoolkit/nncf.git" "onnx<=1.16.1"
第3步:使用 NNCF 下载并导出 Llama 3.2
在 从Hugging Face 导出模型之前,确保你已在此处接受使用协议。
然后,使用以下命令下载并导出模型:
optimum-cli export openvino --model meta-llama/Meta-Llama-3.2-3B-Instruct --task text-generation-with-past --weight-format int4 --group-size 64 --ratio 1.0 --sym --awq --scale-estimation --dataset “wikitext2” --all-layers llama-3.2-3b-instruct-INT4
注:对于从 HuggingFace 网站上下载 Llama-3.2 模型的开发者,如果使用 Windows 系统,可以在 powershell 窗口中首先进行如下设置,再使用上述命令进行模型的下载、转换及压缩:
pip install -U huggingface_hub
set HF_ENDPOINT=https://hf-mirror.com
如果是 Linux 系统,在终端窗口中使用如下命令首先进行设置:
pip install -U huggingface_hub
export HF_ENDPOINT=https://hf-mirror.com
也可以直接在魔搭社区
https://www.modelscope.cn/models/LLM-Research/Llama-3.2-3B-Instruct
进行模型下载。
下载完成后,在上述模型转换压缩命令中将模型名替换为下载后的模型保存的路径,即将命令修改如下:
optimum-cli export openvino --model /Llama-3.2-3B-Instruct --task text-generation-with-past --weight-format int4 --group-size 64 --ratio 1.0 --sym --awq --scale-estimation --dataset “wikitext2” --all-layers llama-3.2-3b-instruct-INT4
第4步:运行模型
你现在可以使用 OpenVINO™ 运行模型推理。运行以下命令:
python chat_sample.py ./llama-3.2-8b-instruct-INT4
默认情况下,示例在 CPU 上运行。要切换到 GPU,只需在 chat_sample.py 中更新 device 参数:
pipe = ov_genai.LLMPipeline(model_path, "GPU")
最后,在我的AI PC集成显卡以及英特尔ARC A770独立显卡上运行推理的情况!
查看视频:https://blog.csdn.net/inteldevzone/article/details/142629530
结论
使用 OpenVINO™ 在本地运行 Llama 3.2 为开发人员提供了一种强大且高效的解决方案,能够最大限度地提升 Intel 硬件上的 AI 性能。通过这种设置,你可以享受更快的推理时间、更低的延迟和更少的资源消耗——所有这些只需最少的设置和编码工作。希望本指南能帮助你快速有效地开始。祝编码愉快!